Hey friends! I’m Akash, partnering with founders at the earliest stages at Earlybird Venture Capital, .
Software Synthesis is where I connect the dots in software strategy. You can reach me at akash@earlybird.com if we can work together.
Current subscribers: 4,710
In recent months, a consistent request from AI application founders I’ve spoken to is a request for red teaming solutions that can improve the robustness of model outputs. The potential productivity and efficiency gains from AI can be entirely offset by the cost of bad actors eliciting model outputs that are harmful, malicious or reveal sensitive information.
An additional tailwind is regulation. The EU AI Act has gone into force this month and providers of general purpose models with ‘systemic risk’ must have:
Where applicable, a detailed description of the measures put in place for the purpose of conducting internal and/or external adversarial testing (e.g., red teaming), model adaptations, including alignment and fine-tuning
Last week, Protect AI announced a new funding round immediately after announcing the acquisition of SydeLabs to expand their AI security suite into red teaming.
“The acquisition of SydeLabs extends the Protect AI platform with unmatched red teaming capabilities and immediately provides our customers with the ability to stress test, benchmark and harden their large language models against security risks.” Protect AI CEO Ian Swanson
Red teaming has long been a cornerstone of security, originating from the military during the Cold War before finding traction in information security. Organisations would enlist ethical hackers to simulate adversaries, searching for vulnerabilities that malicious actors might exploit. These hackers would launch attacks to breach firewalls, exploit software vulnerabilities, and social engineer their way past human defences, with the goal of finding and fixing vulnerabilities.
There’s a rich literature on adversarial attacks on ML models, but LLM red teaming is still nascent.
There are several types of adversarial attacks, with one axes being the degree to which the approach relies on humans or models.
Scale AI, for example, places greater emphasis on human red teams, with automated model red teaming playing a smaller role.
Although Anthropic used national security experts for national security harm red teaming, they relied on model red teaming, to align Claude models to their Constitutional AI framework.
Red teaming is a category at the intersection of AI security and LLM Ops that is becoming increasingly important to safely deploying AI in the enterprise, for both internal (e.g. leaking sensitive information) and external (e.g. harmful, criminal outputs) use cases.
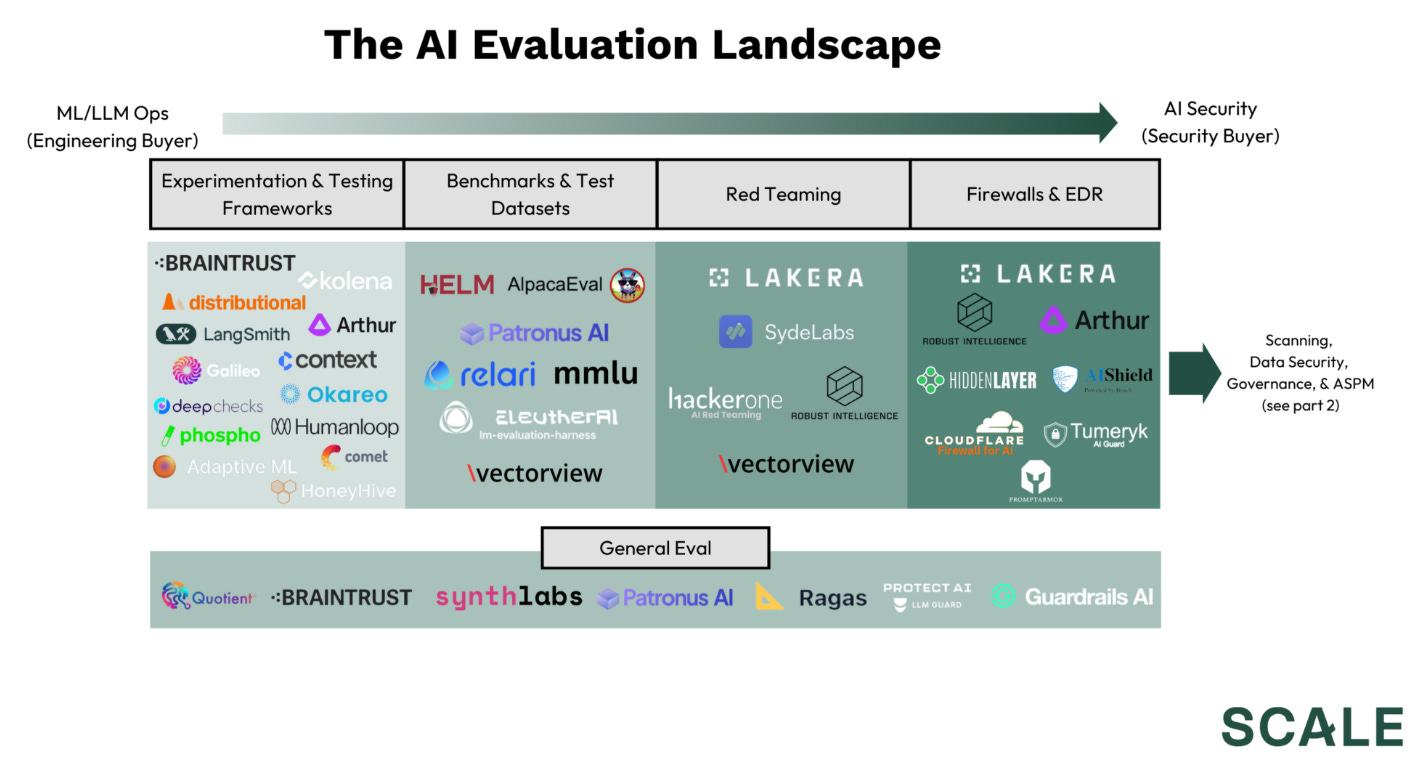
Model-based red teaming entails a red-teamer model attacking a target LLM to trigger unsafe responses. The main challenge with model-based red teaming is that they still require humans to determine if the ‘judge’ model’s evaluations are correct. In any case, human red-teaming is hard to scale and requires lots of training (i.e. Scale AI), given the sheer combinatorial complexity of natural language making it difficult to ensure comprehensive coverage.
Automated red-teaming as a problem has attracted great teams like Haize Labs (as well as the others in the market map above), who have been devising innovative approaches to balancing the tradeoff between robustness and model performance. They highlight approaches such as Latent Adversarial Training (edit the model’s features, as we discussed here), oversight models, uncertainty quantification, and more. The team also released a Red Teaming Resistance leaderboard together with Huggingface.
The key takeaways from exposing a variety of models to various adversarial datasets:
Closed source models are more robust than open source models, but it’s not easy to determine if this is inherent to these models or due to more safety components
Models are most vulnerable to jailbreaks that induce Adult Content, Physical Harm, and Child Harm
Models tend to be very robust to violating privacy restrictions, providing legal, financial, and medical advice, and campaigning on behalf of politicians
Extant mitigation strategies have tended to dilute model performance, which is why we’ll undoubtedly see more teams tackling automated red teaming to safely deploy AI in the enterprise without the associated trade-offs.
For further reading, listen to Ian Webster on the a16z AI podcast, read Lillian Weng’s post, and more from Lakera, Robust Intelligence.
Charts of the week
Coatue’s excellent report ‘Robotics won’t have a ChatGPT moment’ distils the key trends to follow in the stepwise adoption of robots across verticals
Sapphire Venture’s semi-annual State of SaaS Capital Markets review tells a story of continued rotation into efficiency over growth and a more protracted recovery
Curated Content
Evolution of Databases in the World of AI Apps by
Choosing the Right Sales Comp Plan For Your First Enterprise Reps by
Why did BlackRock spend $3.2bn on Preqin? by
Startup Ideas by Conviction Capital
Quote of the week
‘The key here is that investors seem to have forgotten that MDB didn’t win because they had the best document database, but because of the developer ecosystem, capabilities built around it, ease of use, and vendor integrations. The same should apply to Gen AI native apps, vendors aren’t going to win just because they have the best vector search capability.’
Thank you for reading. If you liked it, share it with your friends, colleagues, and anyone that wants to get smarter on startup strategy. Subscribe below and find me on LinkedIn or Twitter.