Margin Wars in AI: Nvidia's Interchange Rate
The perennial incentive to commoditise complements
Hey friends! I’m Akash, an early stage investor at Earlybird Venture Capital, partnering with founders across Europe at the earliest stages.
I write about software and startup strategy - you can always reach me at akash@earlybird.com if we can work together. I’d love to hear from you.
Current subscribers: 4,280
We recently discussed how innovations in hardware and model capabilities are driving precipitous declines in training and inference costs, as well as order of magnitude improvements in latency. The developments continue like a rolling thunder.
If we take a step back and examine the arc of this platform shift, determining how value will accrue remains a key question.
wrote an excellent piece on how value accrual in AI may mirror the arc of mobile and the cloud cloud, where initial value capture by the lowest layer of the stack (semis) is eventually eclipsed by the applications closest to the end consumers.The march to this inversion will be assisted in no small part by the strategic incentive to commoditise complements.
The goal of every company is to build a moat around one part of a value chain, and then commoditise their complements. This both prevents competitors from arising and also enhances the overall value of their offering, both to their customers (because the overall cost of the value chain is lower because commodity providers compete prices down) and to themselves (because they capture the majority of the value in the value chain).
Ben Thompson
Joel Spolsky wrote the seminal post on how this incentive motivated Big Tech initiatives to fund open source projects. Lowering the cost of a complement drives up demand for the associated software product. This has been the impetus for the open sourcing of software like Android, PyTorch, TensorFlow, and now Llama.
I was arguing for this idea of trying to increase willingness to pay. One of the ways to do that is if you have a complementary good or service to complement. The idea in economics is if the cost of your complement goes down, the value of your good or service goes up.
What Google did with Android is thinking like what was their good or service they're trying to sell, what was their complement?
And right, so for them, essentially, they're like, we're going to give away the complement, so we're going to make our complement basically free, which allows the willingness to pay to go off for what we care about, which is advertising. So in the spend on mobile. In a sense there, it was a very interesting strategy in the context of that whole willingness to pay increase the value of your good or service, get your complement, drive it to zero
Nvidia’s Interchange Rate
In the AI stack, the complement commoditisation incentive runs right down the value chain, from applications to tooling, to the research labs and the compute providers. The incentive is eminently clear in the case of Meta:
To put it another way, Meta’s entire business is predicated on content being a commodity; making creation into a commodity as well simply provides more grist for the mill.
Meta is uniquely positioned to overcome all of the limitations of open source, from training to verification to RLHF to data quality, precisely because the company’s business model doesn’t depend on having the best models, but simply on the world having a lot of them.
Another exhibition of this are the recent releases of frontier open source models from Snowflake and Databricks; both companies are seeking to capture the residual value of enterprise AI adoption.
Though Wall Street hasn’t been kind to announcements of increased capex, it’s clear that the amount of capital being dedicated to open source model development will push us into an abundance of models tailor-made for specific modalities, use cases and different Pareto efficiencies (cost vs latency vs accuracy).
At this stage of the platform shift, margins increase as you go up the stack from semi and compute layer (e.g. Nvidia 85% margins) to their nadir at the application layer (where they are c. 50-60%).
Daniel Gross articulated the incentive for each layer above Nvidia to cut costs as:
It’s sort of like how much would you be willing to architect your infrastructure as a fintech company if you could lower your interchange rate? Well, the answer is usually a lot and the Nvidia margin is a kind of interchange rate for tokens, and you’re very much willing to do the work and the schlep for custom architecture if it works in a way that people just weren’t willing to do in 2017 because very few companies had revenue coming in.
From compute up to applications, the incentive to commoditise complements is multi-fold: fortify moats, increase demand, and increase margins.
The Margin Wars
The great team at
have spoken a lot about the four wars in AI: Data quality, GPU rich vs poor, Multimodality, and RAG/Ops war.There are margin wars underway too.
Application builders are in the business of building great experiences - as Aravind of Perplexity put it:
One thing I would say, very inspired by Jeff Bezos is the end user doesn't care what models you're using or what indexes you're using. All they want is a great product experience.
Application builders will expand margins by building great experiences that abstract away the complexity of agentic design patterns, RAG, model routing, guardrails, prompt augmentation, and all of the remaining surface area that LLMOps companies are building on. But they’ll also benefit from Wright’s Law.
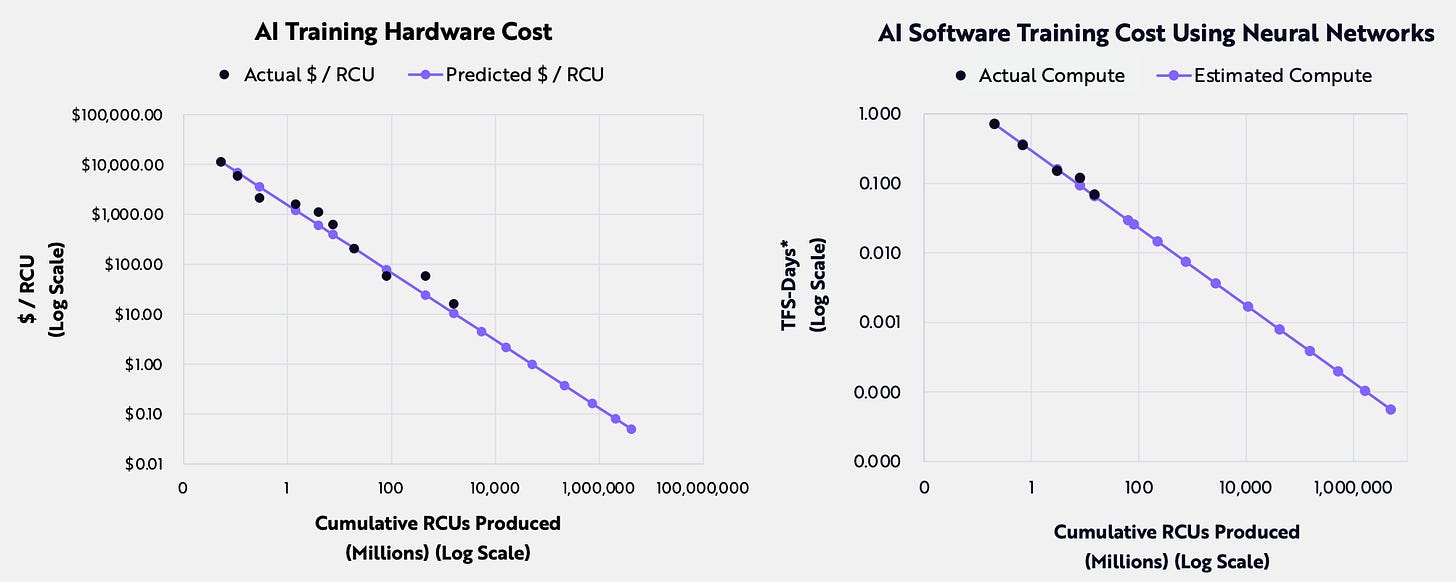
Training at very cutting edge will continue to be the province of a handful of companies, but the collapsing of training and inference costs for each generation of frontier models makes a compelling case for margin expansion at the application layer.
Scaling laws dictate that the Nth generation of models will ravenously consume expensive compute
Whilst costs collapse rapidly for N-1 models
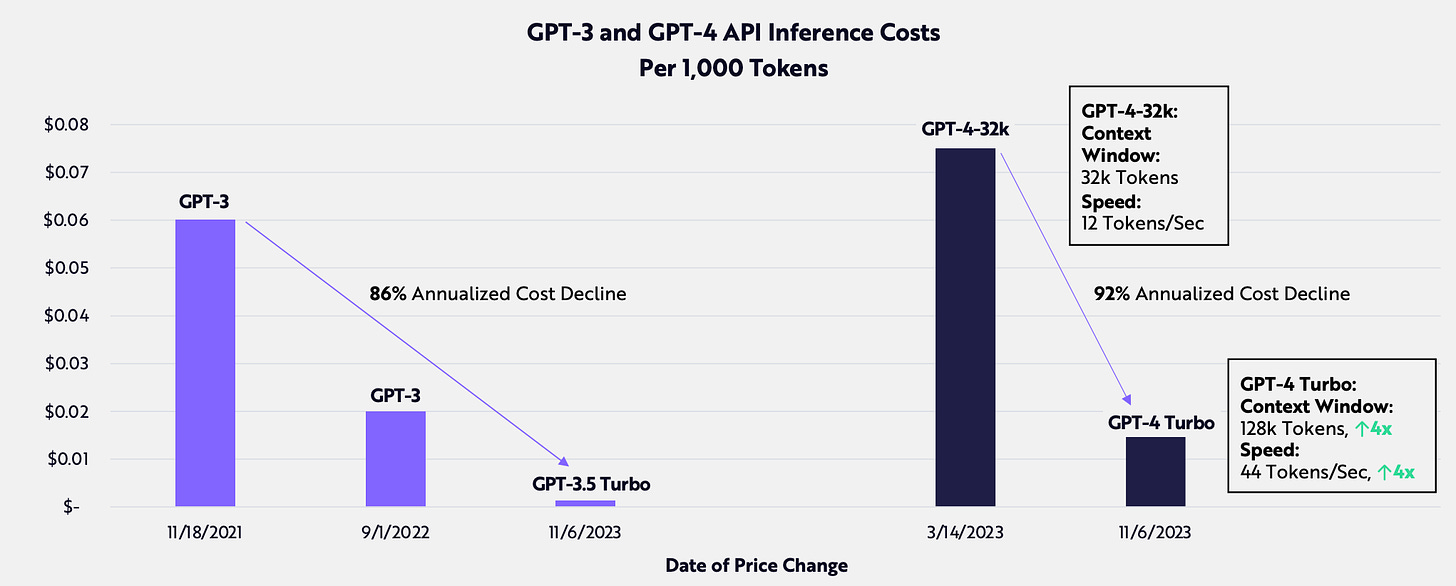
Application builders have been deftly sequencing their usage of models: deliver 10x better experiences with expensive frontier models in the immediate period after release at diluted margins, before slowly graduating to higher margins as the inevitable cost decline ensues.
In parallel, enterprises are increasingly repatriating public cloud workloads, given that repatriation can drive as much as 50% savings.
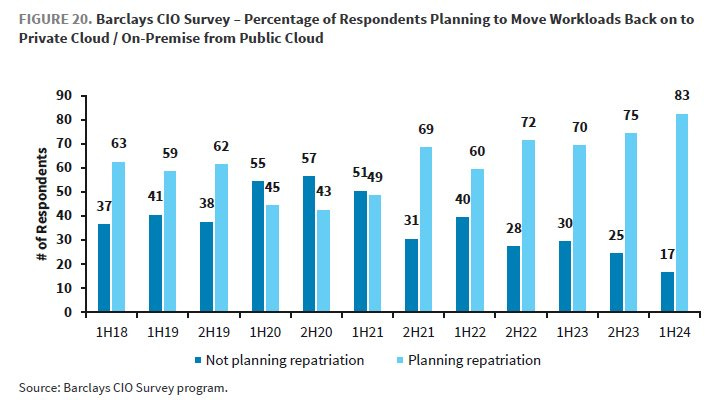
As Apoorv points out, we’re still in the first innings and have a long way to go, but the paths to margin expansion and compression are becoming visible.
Charts of the week
‘Mountain Climbers’ in security like PANW have overcome historical doubts about value accretion through M&A, increasing product scope inorganically
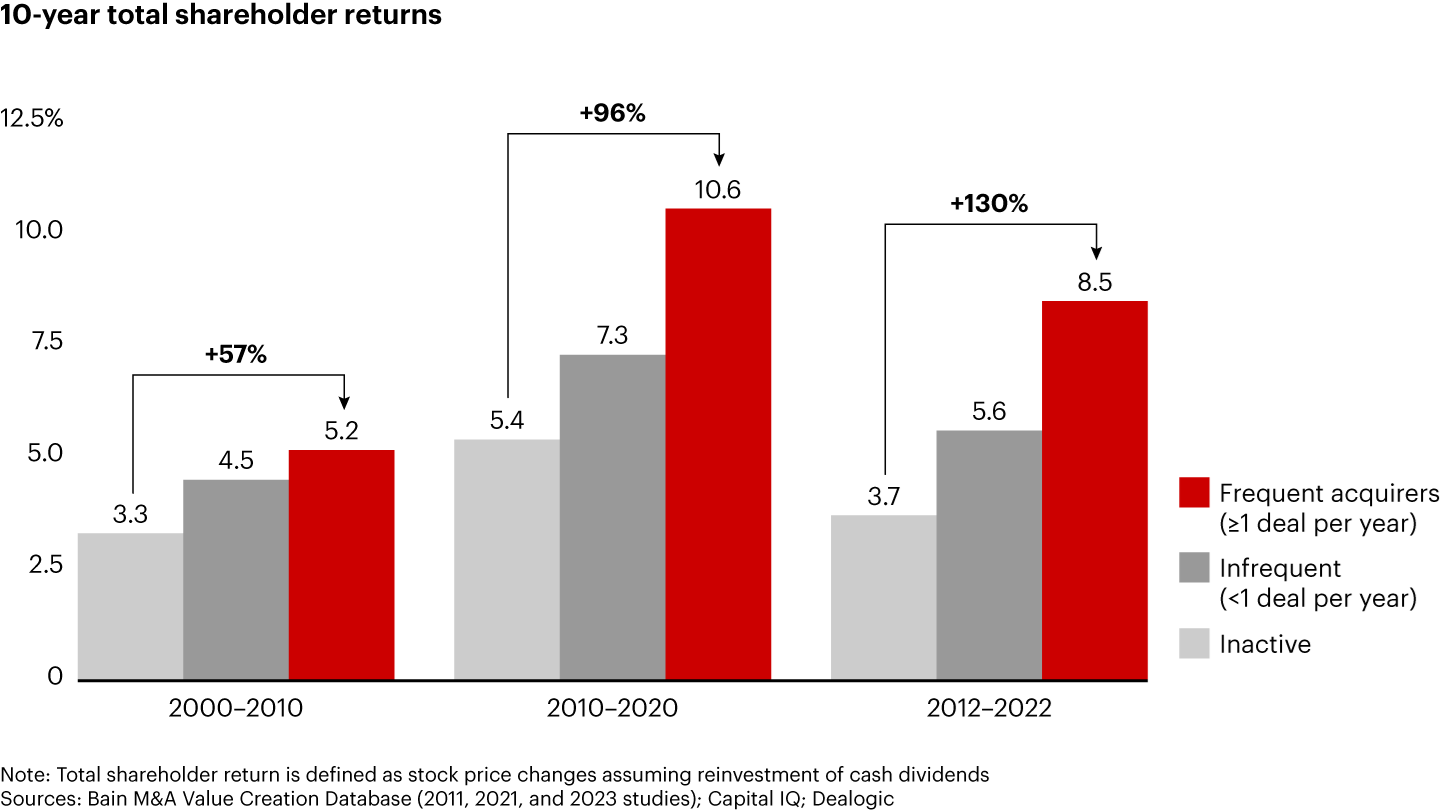
Model proliferation results in buyers becoming more sophisticated in evaluations across a number of dimensions
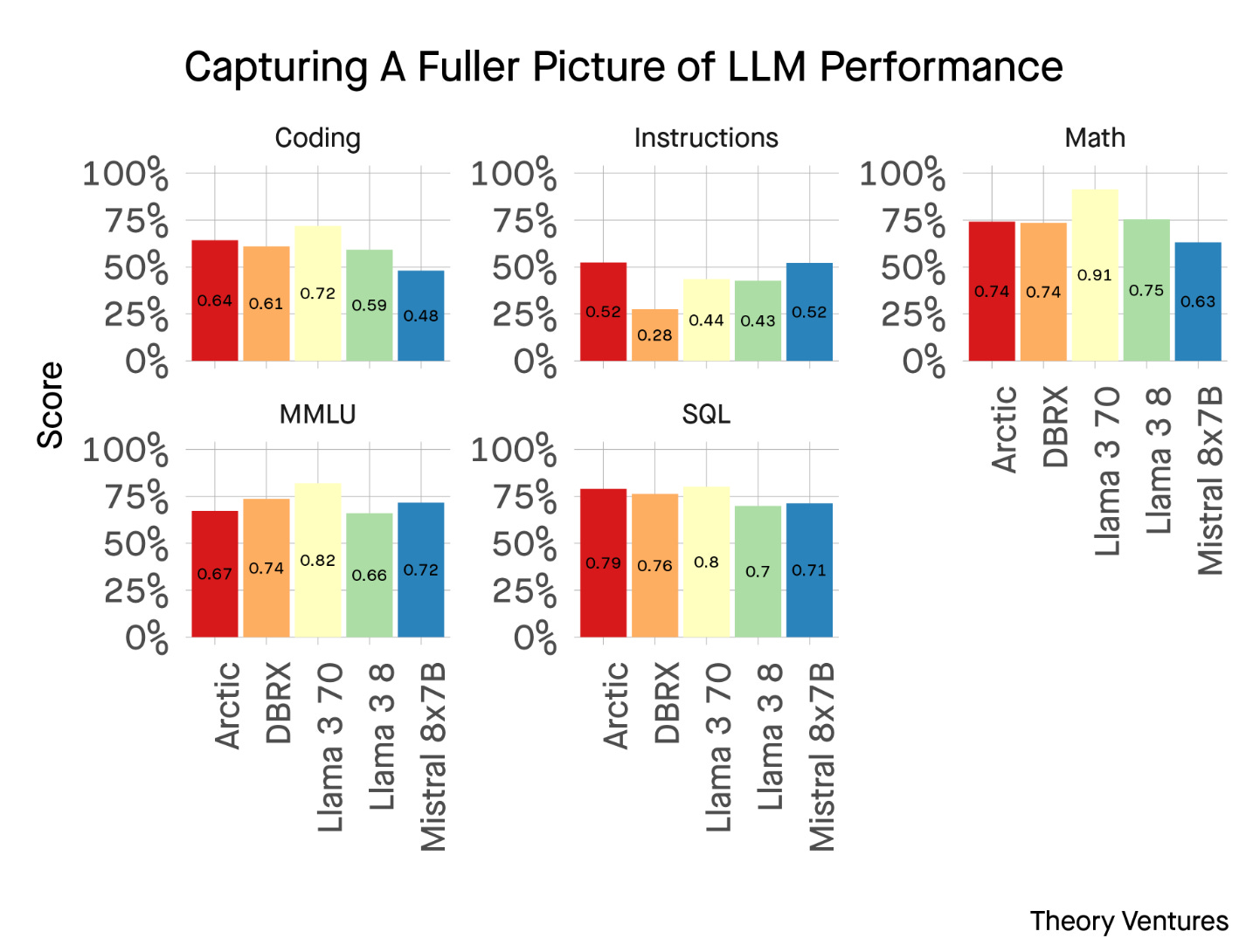
Sweden’s capital markets are a model for the rest of Europe, where equity market participation is significantly higher among retail and institutional investors
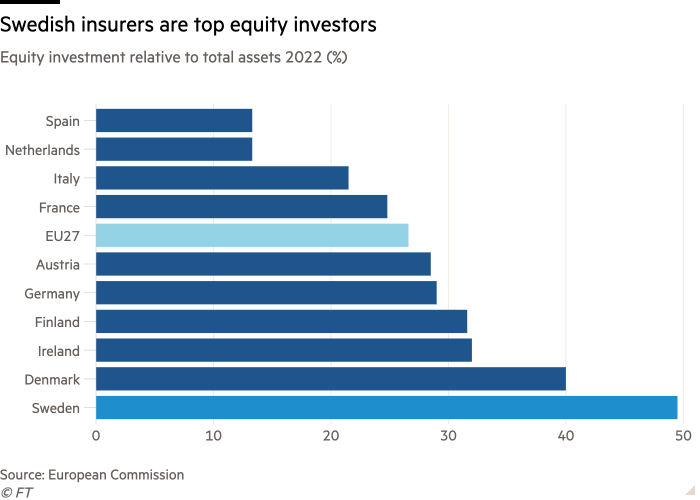
Reading List
Quotes of the week
‘With the MMLU general knowledge (high school equivalency) score now asymptoting, buyers of models will begin to care about different attributes.
For a data-focused customer base, SQL generation, code completion for Python, & following instructions matter more than encyclopedic knowledge of Napoleon’s doomed march to Moscow.’
Thank you for reading. If you liked it, share it with your friends, colleagues, and anyone that wants to get smarter on startup strategy. Subscribe below and find me on LinkedIn or Twitter.
thanks for the shoutout!
Love ❤️ this. Thank You 🙏