Second-order effects of model and hardware improvements in AI
Novel paradigms unlocked by infrastructure development
Hey friends! I’m Akash, an early stage software & fintech investor at Earlybird Venture Capital, partnering with founders across Europe at the earliest stages.
I write about software and startup strategy to help founders & operators on their path from inception to PMF and beyond.
You can always reach me at akash@earlybird.com - I’d love to hear from you.
Current subscribers: 4,240
We’re some way into the ‘sell work’ paradigm of AI-infused software. As AI melds with services, the atomic unit of output (and pricing) will be outcomes.
This concept is symbiotic with the developments of the hard technologies underneath it, reflexively breaking new ground as we climb exponential curves in model capabilities, inference costs, context windows, and latency.
With B200s, we need 1/4th the power to train a 1.8 trillion parameter GPT-MoE model.
Inferencing performance of Blackwell chips could improve from 5-21x for a ~2x increase in compute costs. Clark Tang
This year, I have very, very credible sources all telling me that the average tokens per second right now, we have somewhere between 50 to 100 as like the norm for people.
Average tokens per second will go to 500 to 2,000 this year from a number of chip suppliers that I cannot name. Shawn Wang, swyx
This is a general trend we have observed a couple of years ago. We called it Mosaic's Law where a model of a certain capability will require 1/4 the [money] every year from [technological] advances. This means something that is $100m today goes to $25m next year goes to $6m in 2 yrs goes to $1.5m in 3 yrs. Naveen Rao
‘This upgrade brings a massive 1 million-token context window, which is the largest in the industry. This allows customers to perform large-scale changes across your entire code base, enabling AI-assisted code transformations that were not possible before’. Brad Calder
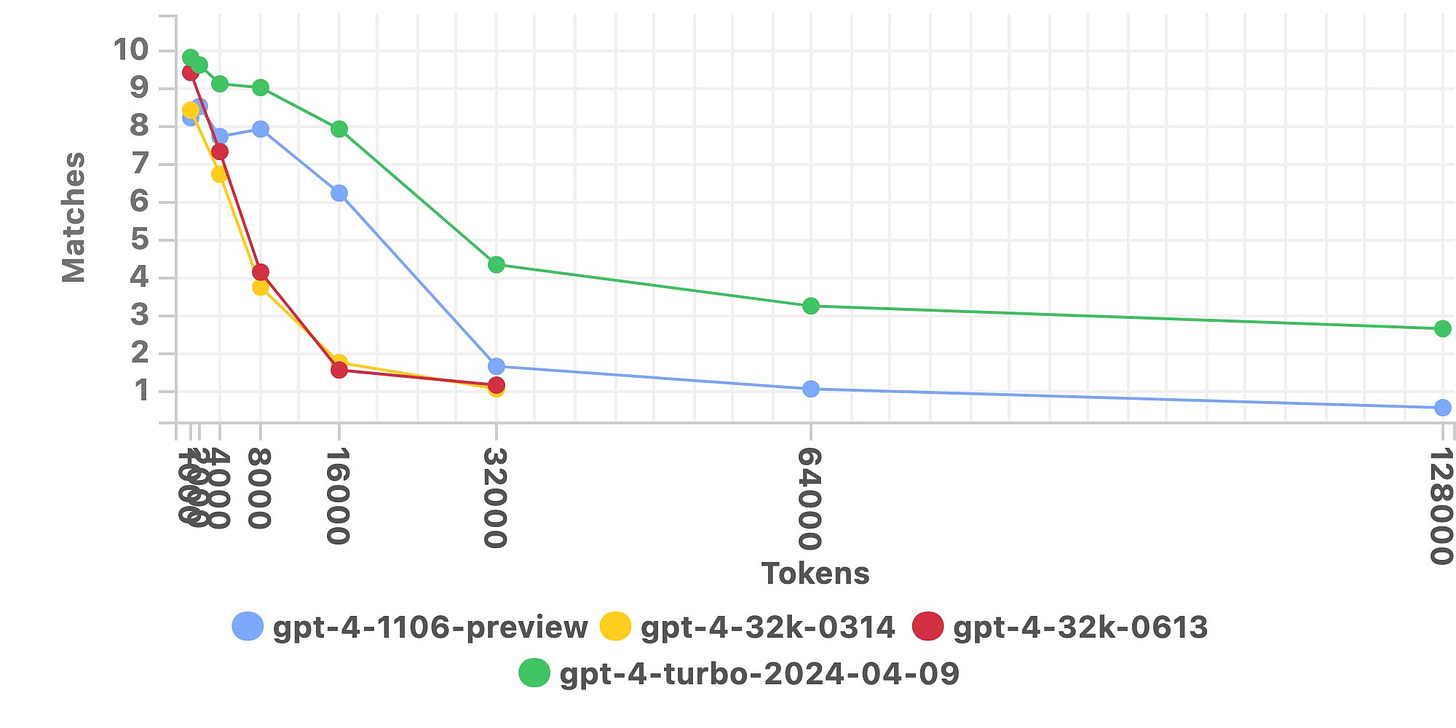
Inference costs are spiralling down and performance will improve disproportionately compared to cost.
Tokens per second are going to increase by an order of magnitude.
Context window size and utilisation have increased several folder in one year.
The cost training base models from scratch is going to fall by 75% every year.
If we combine all these infrastructure improvements, what possibilities are unlocked?
We’ve all seen the Groq demo, but the true latent value unlocked by astonishingly cheap and fast inference are difficult to overestimate.
It’s a user interface unlocker too. With slow model outputs, you were forced to have this streaming tokenization, the stream of tokens basically coming at you and now with speed, speed has always been a feature and I think actually in many ways this is just a reminder of a perennial rule of user interface design, which is that speed matters, latency matters.
It also feels more superhuman in a way, because you can get a whole essay in seconds and you can get a book in minutes and there’s a way in which the superhuman feeling is stronger, but also I think you could have the model, for example, if you’re willing to spend the money, it’s more reasonable to have the model explore several paths and maybe it’s going to try ten things and pick the one that works best because it can do it very quickly.
So some of the user interface hacks that we’ve gotten used to are Bing, for example, outputting some text and then deleting it and saying, “Sorry about that, I said something I shouldn’t have said,” or whatever. At a slow speed it’s almost comical, it’s just this really strong sense that we’re in this very early stage of the development, but at speed it might not even be noticeable so I think it just unlocks a whole bunch of new experiences that have not been possible before and that makes it exciting.
Andrew Ng has written about the incredible potential of agentic design patterns and the performance gains they can drive; GPT-3.5, wrapped in an agent loop, achieves up to 95.1% on the HumanEval benchmark, far surpassing the incremental performance gains on zero-shot prompting between GPT-3.5 and GPT-4.
What are the implications of collapsing inference cost and speed (not to mention larger context windows with better utilisation) on agent design patterns like reflection, tool use, planning and multi-agent collaboration?
If AI is like energy, as Sam Altman has argued, then elasticity will inevitably result in higher consumption of agent-enhanced models - which will in turn continuously drive productivity gains.
The logical endgame is higher throughput of work, as Alessio Fanelli has suggested.
Alessio made the case that Maximum Enterprise Utilisation, (analogous to Maximum FLOP Utilisation), has historically been constrained by Metcalfe’s law and an inverse correlation between work hours and productivity rates.
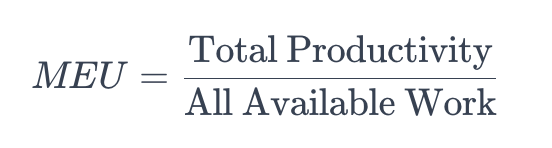
To come full circle, as we enter the age of full-stack employees sold as software, we’re also on the precipice of huge latent demand waiting to be unlocked by improvements in hardware and models that we have an increasing line of sight to.
What is the ceiling on full-stack employees beyond the typical 20-40% MEU of human workforces?
Can the productivity of asynchronous employees working 24/7 surpass the total Available Work in an enterprise?
If so, do we push the boundaries of Available Work that we consider viable and Pareto efficient?
Before we get too abstract, it’s important to underline how important it is for agent-centric startups to incorporate this framework of MEU for the specific function or vertical they’re addressing.
I’ll reserve judgment on AGI scaling laws, but we have clear visibility into hard technological innovations that unlock immense enterprise value.
What a time to be building.
Charts of the week
How CISOs measure ROI against priorities
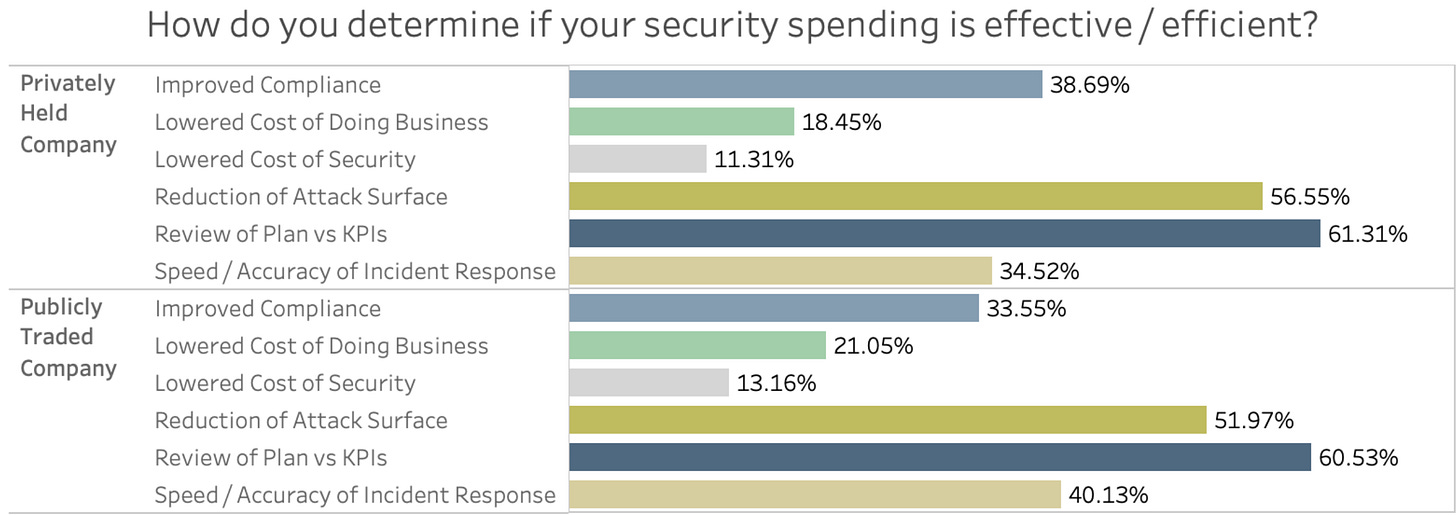
The markets want growth and profitability, but those at smaller scale need to become more efficient to be rewarded
Reading List
Fine porcelain maker Maruwa becomes the hottest bet for cooling AI data centres Financial Times
Why Cash App Pay is a big deal for Block
How Google lost ground in the AI race Financial Times
The Death of Deloitte: AI-Enabled Services Are Opening a Whole New Market Emergence Capital
$10 Billion Productivity Startup Notion Wants To Build Your AI Everything App Forbes
‘Like Wikipedia And ChatGPT Had A Kid’: Inside The Buzzy AI Startup Coming For Google’s Lunch Forbes
Quotes of the week
‘But what’s different about Danny is he seems to do that even when his core beliefs are attacked or threatened. He seems to take joy in having been wrong, even on things that he believes deeply. And so I asked him about that—why and how?
On the why question, he said, Finding out that I was wrong is the only way I’m sure that I’ve learned anything. Otherwise, I’m just going around and living in a world that’s dominated by confirmation bias, or desirability bias. And I’m just affirming the things I already think I know.
On the how part, he said for him it’s about attachment. He thinks there are good ideas everywhere, and his attachment to his ideas is very provisional. He doesn’t fall in love with them, they don’t become part of his identity.
He had that ability to detach and say, look, your ideas are not your identity. They’re just hypotheses. Sometimes they’re accurate. More often, they’re wrong or incomplete. And that’s part of what being not only a social scientist, but just a good thinker, is all about.’
Thank you for reading. If you liked it, share it with your friends, colleagues, and anyone that wants to get smarter on startup strategy. Subscribe below and find me on LinkedIn or Twitter.
Thank you, Akash, for sharing my essay on Cash App!