Hey friends! I’m Akash, partnering with founders at the earliest stages at Earlybird Venture Capital.
Software Synthesis is where I connect the dots on software and company building strategy. You can reach me at akash@earlybird.com if we can work together.
Current subscribers: 4,740
War Times In SaaS
In December 2023 we had the death of hypergrowth software (software companies projected to grow revenue > 40% in the NTM).
Then, in June of this year, we had the death of high growth software (software companies projected to grow revenue >30% in the NTM).
H1 ‘24 earnings tell a story of continued headwinds in software, as Q2 prints dampened expectations of a soft landing and a sustained recovery implied by Q4 ‘23 results. SaaS multiples remain below pre-COVID levels and of the 80% of S&P 500 companies to have reported second quarter earnings, only 48% had a positive revenue beat, the lowest since Q3 2019.
Along with macro headwinds, the markets are telegraphing where we are on the S-curve of software adoption and how many innings of growth lie ahead, as well as which companies are AI market share donors or gainers (closely coupled with discourse on the rise of ‘services as software’ using AI to expand beyond software budgets into much larger services budgets).
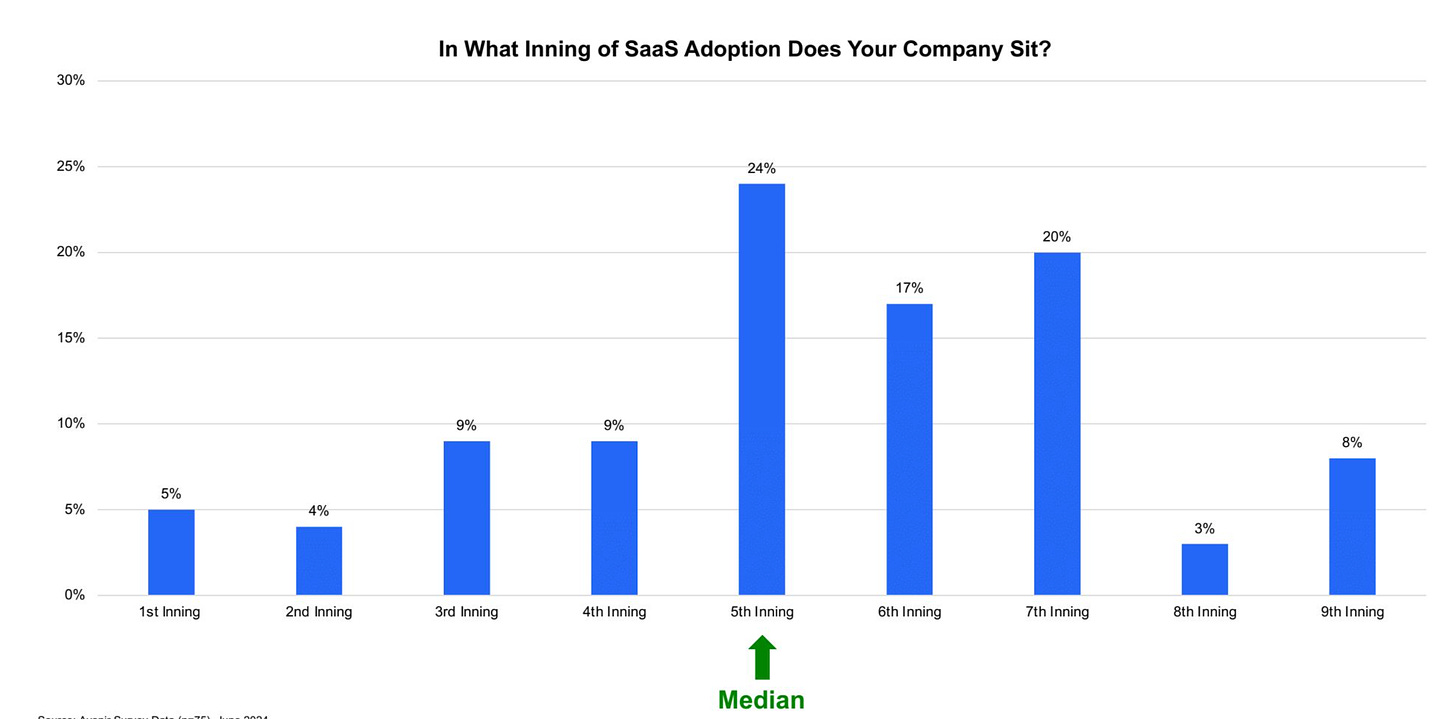
Against this continuous backdrop of belt tightening, AI/ML was one of the only categories of software spend to rise up the agenda for CIOs QoQ, with 16% citing AI/ML as their top priority.
As to whether AI applications have graduated beyond innovation budgets to more predictable software budgets, it’s becoming more evident that the dollars for AI apps are being reallocated from software budgets.
With each quarter we get new directional data on the debate of incumbent vs startup AI spend capture - Microsoft Copilot, Github Copilot, and ServiceNow are but a few examples of prolific uptake of AI SKUs from incumbents.
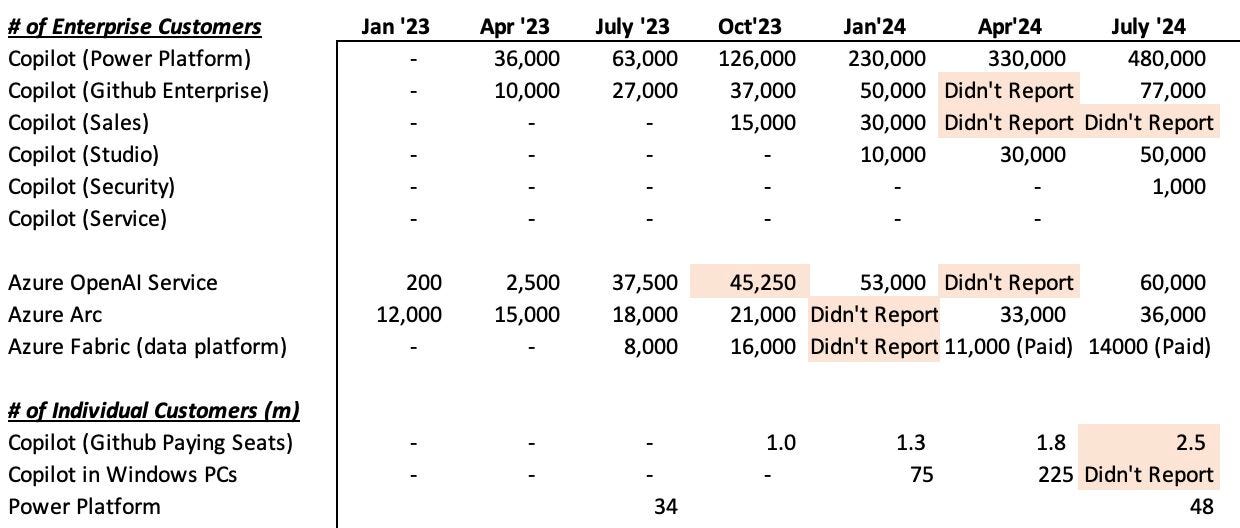
We’re still in the skeuomorphic phase of AI Apps, underestimating the use cases and value creation unlocked by the latent capabilities of transformer models. There’s a range of views on just how radically GUIs and seat-based pricing models will be upended, but there’s more consensus that we’re very early in the apps and infrastructure cycle of AI.
There’s an inverse correlation between the profoundness of an innovation and the time it takes for it to actually make a difference. The Internet took 20 years to your point, for the feed to come along, or 15 years, wherever it might be, which is the feed to my mind is the core Internet sort of innovation. That was something that could not be done before to have a dynamically created list of content that never ends, that is personalized to every single individual, that is fundamentally new. It took 15 years to get to it, and that’s what unlocked the advertising model, it unlocked the entire economy of the Internet. That’s what changed our politics, changed society. It took a really long time, even though in retrospect it’s very obvious — I’m making your social media point in a different way. But it seems like, what were we doing in the intervening 15 years?
The hyperscalers are inclined to overinvest in capex to avoid falling behind in frontier model capabilities. Accenture and other consultants have been booked hundreds of millions in revenue to educate enterprises on AI’s ROI and various use cases. Aside from data walls, assuming we continue to advance frontier model capabilities, where and when will AI be a catalyst for upgrade cycles in software?
AI Catalysing Upgrades
Apple Intelligence is likely to drive a record upgrade cycle for Apple devices. A combination of near all-time high replacement cycles and hardware constraints that limit Apple Intelligence to only 8% of a record installed base are going to significantly contract replacement cycles for iPhones.
AI, like 5G before, is enough of an innovation to spur consumers to upgrade their phones - Apple Intelligence’s success will still rest on other variables like app developers exposing their data to the OS, but even the base case looks promising. We might even see Apple tiering their devices on the basis of RAM, with most 8GB smartphones lacking the sufficient memory to run AI on the edge - if Apple Intelligence becomes the killer use cases many are making it out to be, consumers will pay up.
By contrast, AI’s impact on upgrade cycles across software categories will vary from category to category. IT execs who had gone through a trough of disillusionment with AI once ML applications had been exhausted need to have their AI story together as the markets assess which companies are getting ‘fitter’ off the back of AI cost efficiencies (e.g. Klarna). Startups looking to capture enterprise AI spend will hope that buyers view AI as a catalyst to reexamine the AI credentials of their incumbent software, no matter the cost to rip and replace, and issue RFPs for newer vendors that can uniquely deliver AI outcomes that incumbents are incapable of shipping.
One framing for categories that are likelier to exhibit upgrade cycles is ‘category velocity’ from
.Contrasting payroll software with security, Buck makes the observation that software categories that align importance to the company/executive with upside for the champion tend (high velocity categories) tend to move faster, with market structures in constant flux.
By this estimation, ERP is a lower velocity category. Even though upgrade cycles are shortening, potentially owing to buyers looking for potential AI capabilities in newer vendors, it’s not a category that’s seeing the same acceleration in upgrades as others.
Security, on the other hand, is a high velocity category with the second most resilient IT budget in 2024 and plenty of scope for AI-native vendors to catalyse upgrade cycles for buyers. Transformers can accelerate integration builds, normalise log data, and help process larger and larger swathes of unstructured data (with continuous improvements in context window utilisation and function calling driving enhanced RAG) to unlock further ROI.
The category velocity framing is a useful heuristic for upgrade cycle momentum, as it more or less maps neatly to the positioning of categories based on whether AI is incremental to top or bottom-line impact or a step-change improvement.
If we look at developer tools, the divergence in Stack Overflow and Github Copilot usage tells a tale.
Customer service, a category with significant venture dollars flowing into early stage disruptors, has seen incumbent Teleperformance continue to suffer from buyers seeing AI as a catalyst for upgrade cycles.
Conclusion
The adage that startups must acquire distribution before incumbent gets innovation has been rehashed ad-nauseam in the debate of AI value capture, but the reality is that it’s not even clear that AI apps as a whole have caught up to the level of innovation of incumbents - the early report card on AI App adoption highlights how much room there is to improve.
It’s also why the default posture of enterprises is to consolidate around existing vendors who have data gravity, already own the workflow, and have legions of employees who have been trained on their GUI (lowering TCO).
The onus is on AI-native companies to deliver 10x better outcomes that incumbents will struggle to replicate. At this early juncture, the well-known vectors of counter-positioning are UI/UX and pricing models.
If we extrapolate foundation models into the future, AI-native apps need to also push the boundaries of capabilities in ways that only they can, with the knowledge that some upgrade cycles will have to be annealed.
Charts of the week
team produced a good graphic of the channel mix for PLG and SLG motions as companies scale
The model quality/quantisation trade off visualised by Fireworks, demonstrating that different use cases will require different combinations of latency, cost, performance
A great analysis of hybrid fintech/SaaS business models of leading SMB SaaS vendors
Curated Content
How Fireworks evaluates quantization precisely and interpretably by Fireworks
Fast by Patrick Collison
Growth Channels By ARR Stage: PLG vs. SLG by
Learning To Give A Damn by Altos Capital
Quote of the week
‘If VCs gave a damn, they would be more interested in building special companies than flipping them, just to make money. They would pay attention and not spray and pray their investments, hoping to get lucky.
Also, they would not over-commit by taking on too much money, too many companies and too many board seats. They would capitalize companies responsibly, not according to how much money they had to invest.
Finally, they would take the time to get to know the businesses and people involved. They would focus on developing talent and not rush out to hire mercenaries looking for quick fixes. There are no short cuts. It is critical to make the proper trade-offs between growth, profitability and sustainability.’
Thank you for reading. If you liked it, share it with your friends, colleagues, and anyone that wants to get smarter on startup strategy. Subscribe below and find me on LinkedIn or Twitter.