Hey friends! I’m Akash, an early stage investor at Earlybird Venture Capital, partnering with founders across Europe at the earliest stages.
I write about software and startup strategy - you can always reach me at akash@earlybird.com if we can work together.
Current subscribers: 4,360
What a week in AI.
Most of us had only begun to digest OpenAI’s releases before Google I/O stole at least some their thunder with their own batch of releases. All said, the demos of multi-modality were remarkable and underlined the unparalleled rate of innovation we’re experiencing. Comparisons to Her are inevitable, with it only being a matter of time until highly intelligent, personalised assistants are in all of our pockets (and for Apple’s sake, our devices will have the RAM needed to run these on device).
In the backdrop, the data wars rage on.
Data exhaustion is near
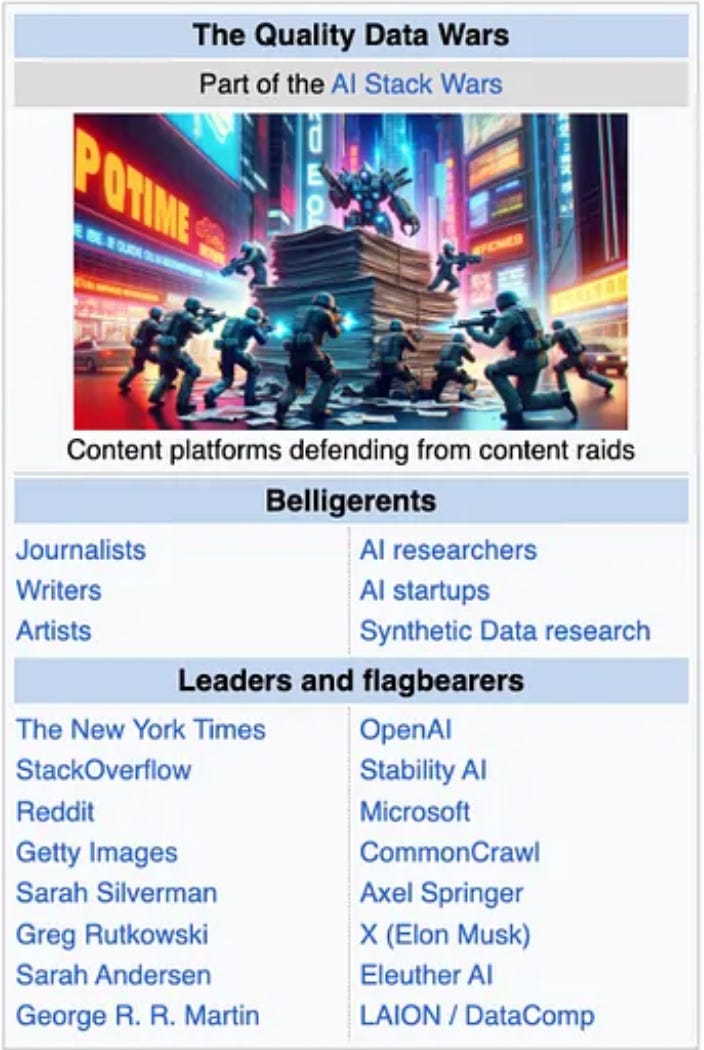
A lot has been said about Llama 3 8B being trained on 15 trillion tokens, well beyond Chinchilla optimal levels. As
wrote:This is a very interesting finding because, as the Llama 3 blog post notes, according to the Chinchilla scaling laws, the optimal amount of training data for an 8 billion parameter model is much smaller, approximately 200 billion tokens. Moreover, the authors of Llama 3 observed that both the 8 billion and 70 billion parameter models demonstrated log-linear improvements even at the 15 trillion scale. This suggests that we (that is, researchers in general) could further enhance the model with more training data beyond 15 trillion tokens.
This affirmation of data scaling laws further accentuates the imminent exhaustion of training data for model training.
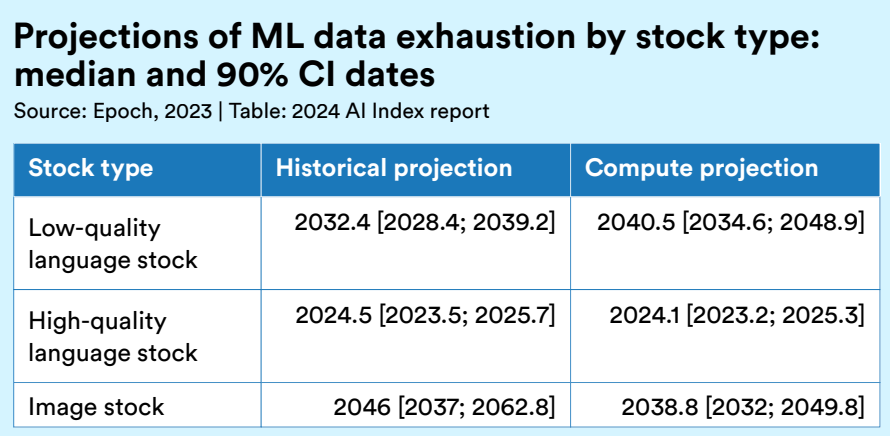
High quality training data is ostensibly behind the impressive performance of many new models, including Microsoft’s SLM, Phi-3. From their technical report:
We follow the sequence of works initiated in “Textbooks Are All You Need” [GZA+ 23], which utilize high quality training data to improve the performance of small language models and deviate from the standard scaling-laws. In this work we show that such method allows to reach the level of highly capable models such as GPT-3.5 or Mixtral with only 3.8B total parameters (while Mixtral has 45B total parameters for example)
Unlike prior works that train language models in either “compute optimal regime” [HBM+ 22] or “over-train regime”, we mainly focus on the quality of data for a given scale.
The availability of data of any kind, high quality or low quality, is depleting rapidly, with implications for the coming generations of LLM/SLMs.
Alexandr Wang rightly pointed out that synthetic data will not be the panacea many would hope for. Relying on synthetic data more than human-generated data results in ‘model collapse’, where models may gradually forget the true underlying data distribution and produce a narrow range of outputs.
Combatting data moats
Given that foundation models have already exhausted most of the data that has ever existed on the internet, there was an inevitability to data partnerships becoming a core GTM function for the research labs.
It’s all the more pressing when you consider the head start enjoyed by Google, Meta and Microsoft over Anthropic, OpenAI and others.
Last week, a deck for OpenAI’s Preferred Publishers Program was supposedly leaked, revealing some of the mechanics behind the deals being struck with content platforms (OpenAI in particular has struck several partnerships recently like Reddit, Axel Springer, The FT and others).
Preferred ‘high-quality editorial partners’ would receive priority placement and ‘richer brand expression’ in chat conversations, as well as more prominent link treatment and financial incentives. In return, OpenAI can train on publisher content and license the content for display in ChatGPT (especially contemporary data which is key given the constant changes in data) - the wider implications of ‘richer brand expression’ and prominent link treatment are for another post.
The capital intensity of data scaling is stark; upon going public, Reddit disclosed that 10% of their revenue came from selling training data for LLMs. Will the advertising business model of the internet be displaced?
articulated the reflexivity between storage/memory cost and data creation as a chain reaction that unleashed the advertising business model (lower cost of memory and storage -> explosion of data generated and stored -> advertisers subsidise the declining storage costs).This chain may be surpassed by the chain reaction of the platforms owning UGC being rewarded by research labs for new training data, for sums that eventually eclipse advertising dollars.
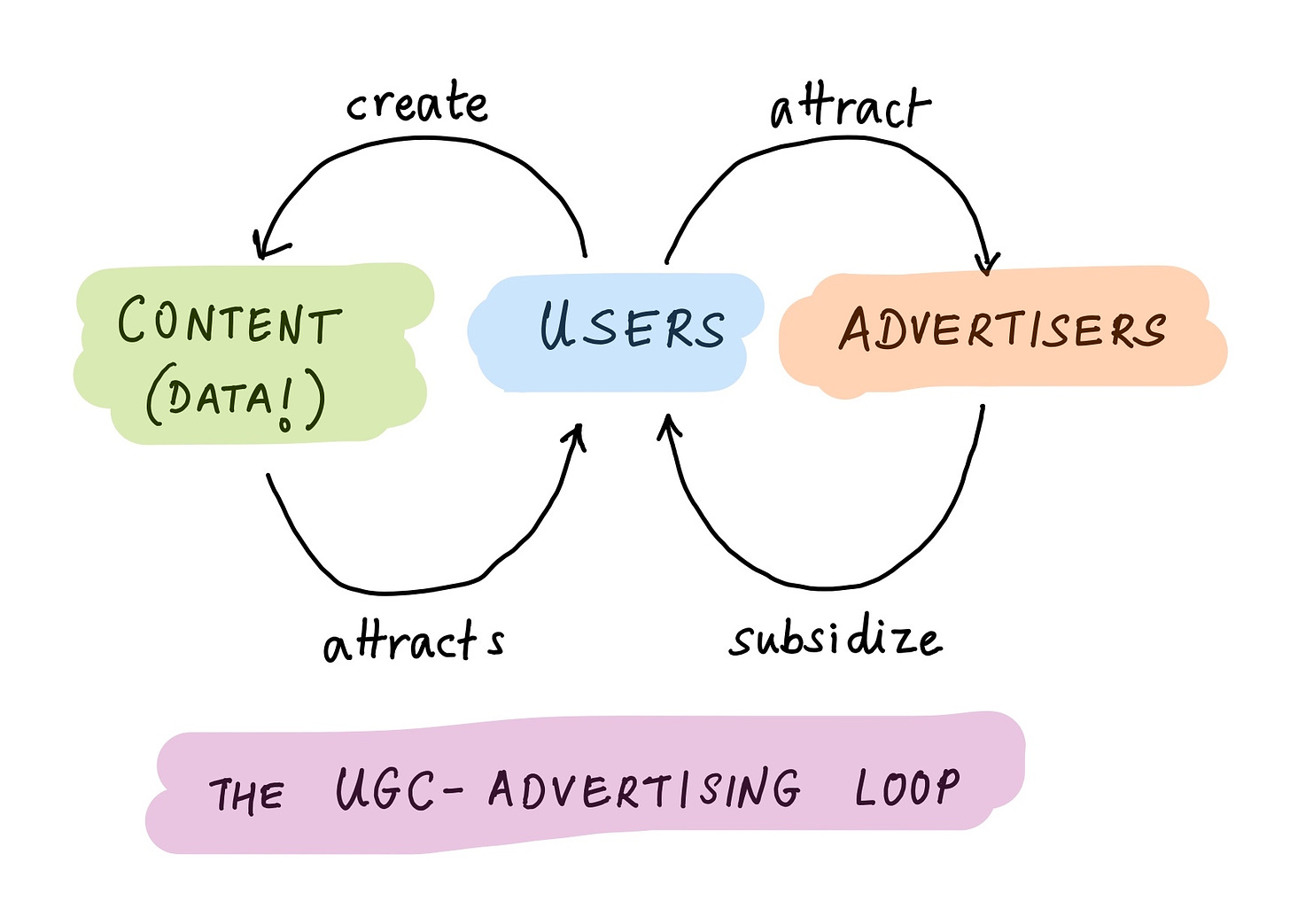
Given how soon we’re going to exhaust high quality public data, the premium for high quality proprietary data is going to appreciate at a rapid clip.
This ought to create new incentives for platforms to prioritise data-hungry research labs over advertisers, affecting product design and UI to optimise for content creation over Return On Ad Spend.
Again,
has written an excellent primer on pricing data assets (in the AI age):There seems to be no upper limit to how much better models become, the more training data you throw at them.
Sprinkling just a little bit of quality on top of your massive corpus — for example, via simple de-duping — has dramatic effects on model performance. As training sets grow ever larger, it’s often more efficient to do this than to acquire the next token; beyond a certain point, data quality scales better than data quantity.
More generally, the entire data stack needs to be refactored, such that generative models become first-class consumers as well as producers of data.
Quite apart from tooling, there's an entire commercial ecosystem waiting to be built around data in the age of AI. Pricing and usage models, compliance and data rights, a new generation of data marketplaces: everything needs to be updated. No more ‘content without consent’; even gold-rush towns need their sheriffs.
Closing thoughts
When we think of scaling laws, we typically think we’re going to be compute constrained, when in reality we’re careening to the intractable obstacle of data production not being able to keep up with the demand from large research labs.
There has been a lot of attention on data centre capex and energy as a potential rate limiter for frontier model training and inference, but the acuity and urgency of data paucity means it’s worth reflecting on the second-order effects of capital intensive data wars.
The advertising business model might not be upended entirely, but licensing of data will be a material enough new revenue line to merit revisions of product design in service of more than just ROAS.
Charts of the week
The evolution of computing, from mainframes to AI
Gemini is ominously closing the gap on ChatGPT, even before Google weaves it in a full-throttled fashion into its ecosystem
Reading List
AI infra trends by Radnor Capital
Eight Key Lessons for Rocket-Shipping AI Products by
With spatial intelligence, AI will understand the real world by Fei Fei Li
Quotes of the week
‘One of your common guests that I love made a statement that I completely agree with was Jeremy Giffon, who talked about that the only enduring edge in life is psychological as human nature doesn't change. That was like words out of my mouth.
How you manage grit? How you manage your ego? Are you comfortable with low status? Are you comfortable being unconventional? Can you delay gratification? Do you have long-term orientation?
Those are keys to be really successful, but it's so hard to do because it's so contrary to human nature.’
Thank you for reading. If you liked it, share it with your friends, colleagues, and anyone that wants to get smarter on startup strategy. Subscribe below and find me on LinkedIn or Twitter.
Superb insight 👏